2. METHODOLOGY FOR ESTIMATING THE GDP AND JOBS IMPACT OF DIGITAL FINANCIAL SERVICES We estimated the potential GDP impact of widespread digital finance using McKinsey’s proprietary Global Growth Model for each of the seven countries on which we focused in this research: Brazil, China, Ethiopia, India, Mexico, Nigeria, and Pakistan. We chose these seven because they represent a diversity of regions and stages of economic development. We then extrapolated the GDP impact from these seven countries to the rest of the developing world. Finally, using the projected increase in GDP, we estimated the number of new jobs that could be created, using historical data on the relationship between GDP growth and employment growth in each focus country. For each of the seven focus countries, we gathered data inputs for the model using a variety of sources. Economic inputs came from publicly available statistics from the World Bank, International Labour Organisation, GSMA, and national governments. We also used proprietary McKinsey datasets, including the McKinsey Global Payments Map and McKinsey Global Banking Pools. (For a complete list of data sources, see the next section of this appendix.) Using these datasets, we built a conceptual microeconomic model of the impact of digital payments and finance on saving, investment, and productivity based on evidence from empirical academic studies. To refine our input data and assumptions, we conducted more than 150 interviews around the world with senior decision makers from a range of digital finance players including banks, telecoms operators, NGOs, fintech startups, government agencies, and regulators. What is the Global Growth Model? The Global Growth Model (GGM) is a supply-side general equilibrium macroeconomic model that covers more than 100 countries with data from 1960 through 2015. The structure of the model is anchored in the academic literature on economic growth models. In addition to the common growth drivers of capital and labor, our model incorporates unique features such as education, energy, research and development (R&D), openness to trade, and financial system depth as distinct drivers of growth. The model also incorporates the core features of a comprehensive macroeconomic model, including labor markets, monetary and fiscal policy expectations, and international trade and investment flows. The model is estimated using dynamic panel “error correction” equations using simultaneous equation techniques to capture interactions among concurrent variables. Validity of the equations is further tested using instrumental variable techniques to control for biased correlations between simultaneously interacting independent variables. The data underpinning the model are obtained from national governments, authoritative international sources, and datasets such as the Barro-Lee education database that are devised by researchers. These datasets are carefully merged to develop extended time series; where gaps remain, we impute missing data using standard econometric techniques. The production function used in the GGM is an augmented Cobb-Douglas production function with fixed capital, energy consumption, and human capital as distinct factors of production (Exhibit A2). The production function incorporates a nested constant elasticity of substitution function to capture the imperfect substitution between capital and energy. The coefficients on the Cobb-Douglas are estimated using the dynamic error correction approach noted above. The human capital index captures both labor supply and the quality of labor in a country. It is defined as the product of employment, average years of education, and an index of work McKinsey Global Institute Digital finance for all: Powering inclusive growth in emerging economies 95
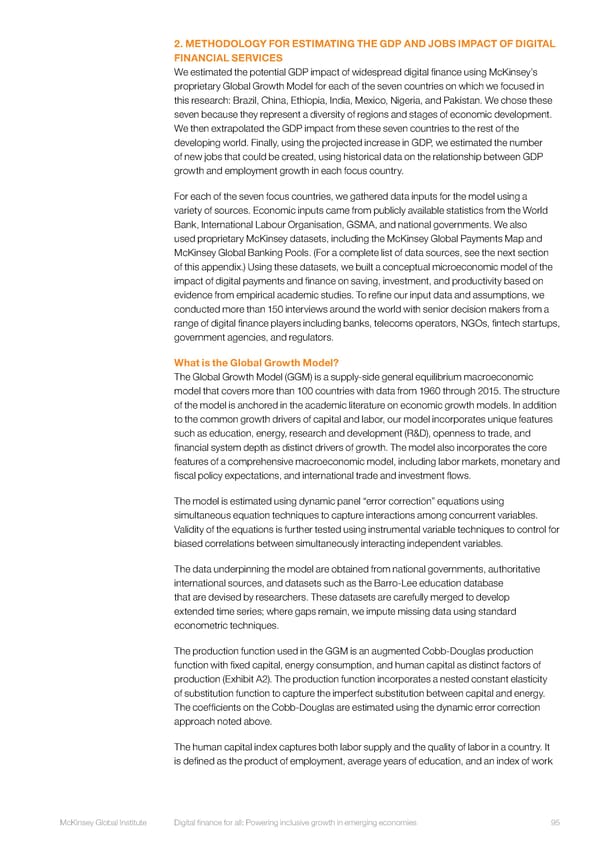